Big data promises a health care remedy
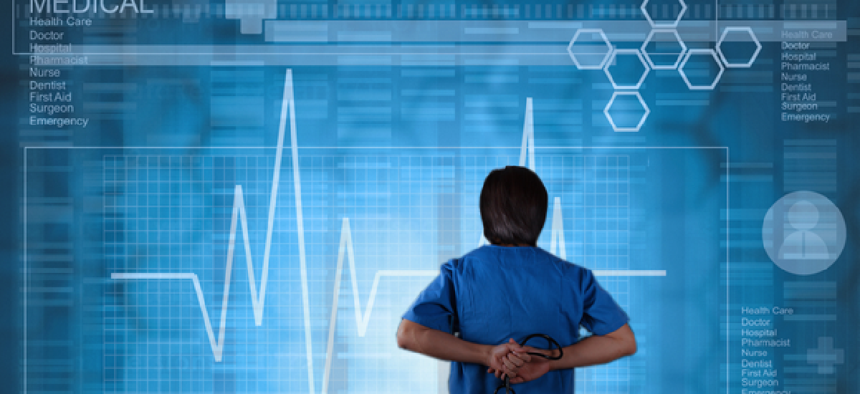
Connecting state and local government leaders
Government agencies are making strides testing uses of big data to predict risks of disease or the path of a killer virus, but hurdles remain, including linking legacy datasets and setting up common vocabularies.
The use of big data to rapidly analyze costs, understand public behaviors and anticipate security threats continues to attract the interest of government agencies that see the technology as a way to gain measurable insights into their most demanding problems.
Nowhere are researchers more active in exploring the uses of big data than in government health care organizations, where data scientists are working toward creating reliable tools for predicting a patient’s risk of disease or a virus’s path of infection.
To some extent health care programs are an obvious target for big data investment. Agencies already have large databases with years of information on diseases and patient health, and they have an urgent need to provide better and more productive information for researchers, doctors and nurses.
The Veterans Health Administration (VHA), for example, has created several big data analytics tools to help it improve health services to its 6.5 million primary care patients.
The VHA’s care assessments needs (CAN) score is a predictive analytic tool that indicates how a given veteran compares with other individuals in terms of likelihood of hospitalization or death. The scores are analyzed by VHA’s patient care assessment system (PCAS), which uses these scores and other data to help medical teams coordinate patient care.
The technology has changed the whole approach at the VHA from being purely reactive to one in which patients at the highest risk of being hospitalized can be identified in advance and provided services that can help keep them out of emergency rooms and other critical care facilities, according to Stephan Fihn, director of the VHA’s Office of Analytics and Business Intelligence.
While still considered fairly rudimentary tools, the CAN score and PCAS demonstrate that big data predictive analytics can work for large populations.
The agency now needs to “markedly ramp that effort up,” Fihn said, and to that end the VHA is working on dozens of predictive models that can be deployed over the next decade. The models will show patients that “this what we know about you, here’s what we think you need,” he said, and be able to do that in a rapid, medically relevant manner.
Big data, open data
Big data tools are also being rapidly developed by the Department of Health and Human Services, a sprawling, 90,000-person enterprise that that both creates and uses data for genomics research, disease surveillance and epidemiology studies.
“There are efforts across the department to try and leverage the data we have,” said Bryan Sivak, HHS’ chief technology officer.
“At the same time a lot of the datasets we maintain, collect, create or curate can be extended to external entities to help them understand aspects of the HHS ecosystem and try to improve on them, such as with CMS (Centers for Medicare and Medicaid Services) claims data,” he said.
One such effort is the OpenFDA project, which essentially took three massive Food and Drug Administration datasets through an intensive cleaning process, Sivak said, and then added an application programming interface (API) so people could access the data in machine-readable ways.
OpenFDA was also linked to other data sources, so that users could access related information from the National Institutes of Health and the National Library of Medicine’s MedlinePlus .
The project, which launched as a beta program in June 2014, has already helped to create “a lot of different applications that have the potential to really help reshape that part of the (HHS) ecosystem,” Sivak said.
Also within HHS, the National Institutes of Health has committed to several big data programs, including its Big Data to Knowledge (BD2K) initiative. The program, begun in late 2013, is aimed at improving researchers’ use of biomedical data to predict who is at increased risk of conditions such as breast cancer and heart disease and to come up with better treatments.
BD2K’s goal is to help develop a “vibrant biomedical data science ecosystem,” that will include standards for dataset description, tools and methods for finding, accessing and working with datasets stored in other locations and training biomedical scientists in big data techniques.
In October last year it announced grants of nearly $32 million for fiscal 2014 to create 11 centers of excellence for big data computing, a consortium to develop a data discovery index and measures to boost data science training and workforce development. NIH hopes to invest a total of $656 million in these projects through 2020.
While physical infrastructure for computational biomedical research has been growing for many years, the NIH said, as data gets bigger and more widely distributed, “an appropriate virtual infrastructure become vital.”
Fundamental challenges
There are significant challenges to applying big data to health care, especially with so many legacy datasets to be integrated and shared. Even the use of the term big data can cause confusion.
“Within agencies there are different definitions and types of big data,” said Tim Hayes, senior director for customer health solutions at Creative Computing Solutions, Inc., and a former HHS employee who worked on data analytics there.
“You need to be sure, when mapping data from one database to another, that you can match various labels that are used. Two different agencies might use the term ‘research,’ for example, but they may not be compatible.”
There are “very arcane differences” between what you would assume are fundamental and consistent definitions that turn out not to be consistent at all, Sivak agreed. “It’s a big problem for sure.”
Another barrier is the lack of data scientists capable of working with and understanding the needs of data analytics programs. The solution starts with recognizing that such people are not IT workers, but occupy a niche all their own.
“A lot of what they do is not working with technology, but is in understanding data,” said Brand Niemann, a former senior enterprise architect and data scientist at the Environmental Protection Agency, who now heads up the Federal Big Data Working Group, an interest group of federal and non-federal big data experts.
The fact is, many agencies may already have people with such expertise on staff but don’t recognize it. It’s a matter of identifying the statisticians that are already working with data, and giving them more of a mandate and outlet to mine the agency’s data, Neimann said.
Get it right, and the results can be transformative.
Accuracy counts
Any analysis of big data has limited usefulness if the information in the dataset is not accurate to begin with. Until only recently, VHA’s Fihn said he had been skeptical that data analytics could reach the levels of accuracy required for clinical use across the VHA. One reason is that, until just a few years ago, the only data available was from health insurance claims.
“In terms of predictive accuracy we use what we call a C statistic,” he said. “A wholly accurate predictive model has a C level of 1.0, and the least accurate has a level of zero. Using (health insurance) claims data, the most accurate level we could get was around 0.65, which is not much better than flipping a coin.”
Between 2010 and 2011, however, the VHA brought online a corporate data warehouse that combined clinical data from some 126 different versions of the VISTA (Veterans Health Information Systems and Technology Architecture) electronic health record the agency had been using since the late 1990s.
With that, Fihn said, and greater availability of data on patient medications and vital signs, predictive models are regularly reaching C levels of 0.85, and are pushing 0.9.
It was a “quantum jump” in terms of the usefulness of predictive analytics, he said, and VHA medical staff feel they can now predict with confidence who the high-risk patients are. And even though predictions are still being published using claims data alone, he said, “for our considerations, we now reject those below C levels of 0.85, and we are actually moving to push things as close as we can to 0.9.”
HHS doesn’t have any global metrics or milestones it wants to reach for big data, Sivak said, though there are specific goals for individual programs. In fact, NIH may have the most expansive set of goals, with BD2K just part of a larger portfolio of activities that NIH is promoting, including cross-agency and international collaboration on big data initiatives and policies.
It’s all a marker for just how quickly minds have changed over big data, Sivak believes. “Back in the day,” nobody would have given any thought to making datasets public or making them available widely within HHS. But over the past five years the value of that has been conclusively demonstrated, he said, “and as a result, the default setting within HHS has changed from closed to open.”
NEXT STORY: Student loan office casts net for ‘creative’ credit analysis